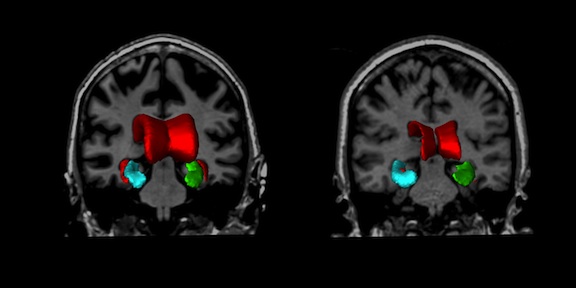
Alzheimer’s Dementia
In Canada, the aging of the population will have significant repercussions, as age is the most important risk factor for developing degenerative dementias, such as clinically probable Alzheimer’s disease (AD). As in most diseases, early treatment of patients, before they have too much irreversible degeneration of brain tissue, is likely to be more effective than later treatment. However, it is currently very difficult to test drugs in patients with early AD, due to the difficulties of making this diagnosis. In addition, only about 10–15% of patients with symptoms of mild cognitive impairment (MCI) suggestive of possible early AD will actually develop the disease per year. European studies in this at-risk group, suggest that ½ of MCIs will not go on to dementia over long-term follow-up. We have developed sophisticated image analysis tools to detect patterns of brain atrophy characteristic of AD, and we have generated statistical models that combine magnetic resonance image (MRI) data with clinical data in order to predict which patients with MCI will rapidly go on to develop clinically probable AD.
Using a large database (n>3000 normal, MCI and AD subjects) of MRI and clinical data obtained through international collaborations, our goal is to properly validate our existing tools for segmentation and image analysis. We will test the general hypothesis that our segmentation and morphology markers (measured on MRI) are valid markers for prodromal AD.
- We will verify the quality of the large MRI and clinical database described below.
- We will validate our MRI-based diagnostic and prognostic tools on a large (n>3000 subjects; 1450 with longitudinal follow-up) database of multi-site MRI data. We will measure the sensitivity, specificity, positive predictive value and negative predictive value of these MRI-based biomarkers.
- We will compare the accuracy of our MRI-based diagnostic and prognostic biomarkers with CSF and PET biomarkers of Aβ accumulation and neurodegeneration. We will show that in the late preclinical / early MCI stage, our MRI biomarkers have greater diagnostic and prognostic accuracy than biomarkers of Aβ accumulation or neurodegeneration. We will show in late MCI and in AD, our MRI biomarkers have greater diagnostic and prognostic accuracy than tests of cognitive or clinical function.
Our prognostic tool will enable enrichment of clinical populations studied in clinical trials, thus making it feasible to perform drug trials in patients with MCI, who in fact, have prodromal AD. Looking forward, the largest impact of these tools will lie in the ability to select patients in the clinic for early treatment without enrolling individuals not likely to progress to dementia over time. We have addressed some of these challenges in our past and current CIHR-, NSERC- and MDEIE-funded research, where we have developed sophisticated automated image analysis and structure segmentation tools to detect patterns of brain atrophy on MRI that are characteristic of AD. We have used these tools to generate statistical models that combine image data with clinical information to accurately predict which MCI patients will rapidly go on to develop clinically probable AD. This study is supported by an operating grant from CIHR.
In the past, we wanted to characterize the spatio-temporal distribution of anatomical changes which are related to AD, MCI and normal aging in the brain and determine if these differences can be used to differentiate MCI from AD. The PCA work developed for TLE was applied to two different data sets, one from Dr. Hampel (Ludwig Maximilian Institute, Munich) and the other from Dr. Chertkow (Lady Davis Institute, Montreal). With the first set, we were able to automatically differentiate normal aging from patients with Alzheimer’s dementia and Mild Cognitive Impairment with high accuracy [4,5]. While this was successful in terms of automated image processing, the result was even more important clinically since this information can be used to corroborate diagnosis and treatment decisions. The procedure was modified to look at the correlation between clinical variables and the morphological principal components extracted by the method. When the PCs evaluated on baseline scans were combined with follow-up clinical data from Dr. Chertkow, we were able to devise a method to predict MCI progression to AD with high correlation almost two and a half years before the subjects presented with clinical signs of AD [6, 7]. This was even more impressive as the data were older and of lower quality that what is normally used today. The potential clinical importance of this work cannot be understated, as there are no techniques based on cross sectional MRI that achieve this level of accuracy in predicting MCI progression to AD. Thus, this method could have a significant effect in decision making for MCI patients. This work was supported by the CIHR POPs grant and resulted in a patent application.
References
[1] Belleville, S., Fouquet, C., Duchesne, S., Collins, D. L., & Hudon, C. Detecting Early Preclinical Alzheimer’s Disease via Cognition, Neuropsychiatry, and Neuroimaging: Qualitative Review and Recommendations for Testing. Journal of Alzheimer’s Disease. 2014.
[2] Eskildsen SF, Coupé P, Fonov VS, Pruessner JC, Collins DL; Alzheimer’s Disease Neuroimaging Initiative. Structural imaging biomarkers of Alzheimer’s disease: predicting disease progression. Neurobiol Aging. 2015 Jan; 36(S1): S23–31.
[3] SF Eskildsen, P Coupé, D García-Lorenzo, V Fonov, JC Pruessner, DL Collins; Prediction of Alzheimer’s disease in subjects with mild cognitive impairment from the ADNI cohort using patterns of cortical thinning. Neuroimage. 2013 Jan 15;65:511–21.
[4] S. Duchesne, J. Pruessner, S. Teipel, H. Hampel, and D.L. Collins, “Successful AD and MCI differentiation from normal aging via automated analysis of MR image features,” presented at Alzheimer’s Association International Conference on Prevention of Dementia, Washington, D.C., 2005.
[5] S. Duchesne, A. Caroli, C. Geroldi, C. Barillot, G. B. Frisoni, and D. L. Collins, “MRI-based automated computer classification of probable ad versus normal controls,” IEEE Trans Med Imaging, vol. 27, no. 4, pp. 509–20, 2008.
[6] S. Duchesne, K. De Sousa, C. Bocti, H. Chertkow, and D.L. Collins, “Predicting MCI progression to AD via automated analysis of T1 weighted MR image intensity,” presented at Alzheimer’s Association International Conference on Prevention of Dementia, Washington, D.C., 2005.
[7] S. Duchesne, Bocti C, De Sousa K, Frisoni GB, Chertkow H, Collins DL. Amnestic MCI future clinical status prediction using baseline MRI features. Neurobiol Aging 2008.